Talk
Abstract
Achieving athletic intelligence in robots is one of the grand challenges of robotics and artificial intelligence. It is the pursuit to enable dynamic control of robots and produce motions that look very natural to their biological counterparts. The aim of this lecture is to provide a holistic view of athletic intelligence which combines three important insights. First, taking inspiration from analytical mechanics (in particular Lagrangian formulation), it will be shown how our universe is a natural optimizer and how our laws of classical physics are a result of this optimization process. Second, building dynamic and energy-efficient robots requires an optimal design where its natural dynamics are in harmony with the task’s dynamics. Third, it will be argued that optimal design is not enough alone, we also need optimal control (for e.g. reinforcement learning) which exploits the dynamics of the robot instead of canceling it out. It will be shown how combining these three insights has led to some of the most advanced athletically intelligent robots.
About the Speaker
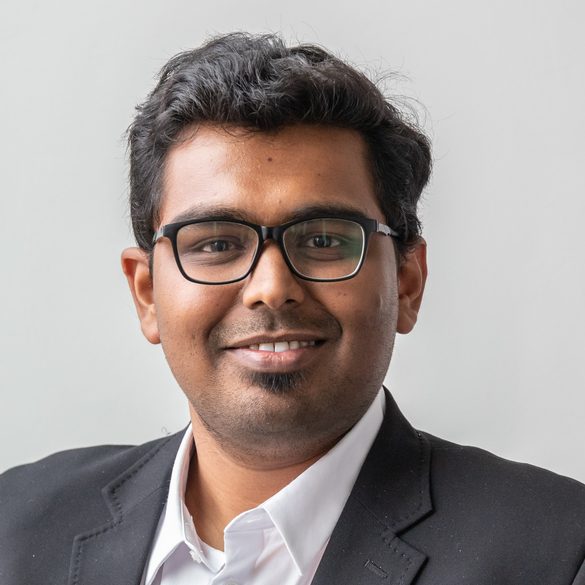
Shivesh Kumar
Researcher at DFKI
Biography
Shivesh Kumar is a Senior Researcher and Team Leader of the Advanced AI Team: Mechanics & Control at the Robotics Innovation Center, German Research Center for Artificial Intelligence (DFKI GmbH), Bremen. He also leads the underactuated robotics lab there. He obtained his PhD degree (Dr. rer. nat.) from Faculty of Mathematics and Computer Science at the University of Bremen in November 2019. Previously, he holds a Masters degree in Control Engineering, Robotics, and Applied Informatics with specialization in Advanced Robotics from Ecole Centrale de Nantes, France in 2015 and Bachelors degree in Mechanical Engineering from National Institute of Technology Karnataka, India in 2013. His research interests include dynamic locomotion, legged robotics, athletic intelligence etc. He also serves as an associate co-chair of IEEE Robotics and Automation Society (RAS) Technical Committee on Model Based Optimization for Robotics.